© 26 January 2023 by Michael A. Kohn
Link to the pdf if this article
Introduction | Coin Problem | Bayes’s Rule: History | Bayes’s Rule: Derivation | Billiards Problem | Endnotes | References
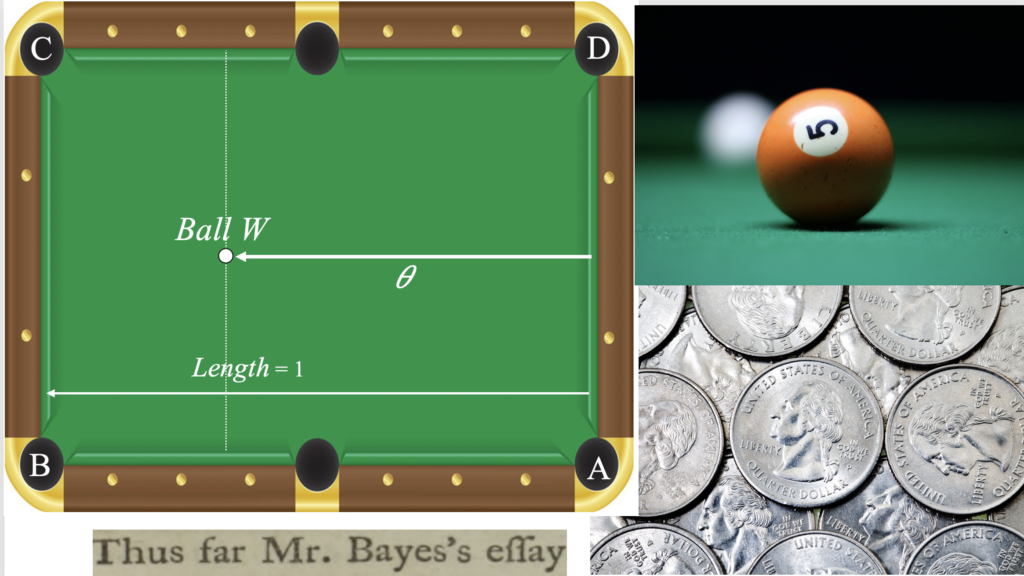
Introduction
You may be confused about the debate between Frequentist and Bayesian statistics over how to use new data to judge a hypothesis. You may also know the formula that updates the probability of a hypothesis based on the likelihood of the observed data under the hypothesis. Since this formula is called Bayes’s Rule, you might think Bayes wrote it; he didn’t. You might think the disagreement is about the formula’s validity; it isn’t.
The debate is about whether we can judge a hypothesis based only on how likely or unlikely the observation would be if the hypothesis were true. Frequentisits say yes. Bayesians say no, we must consider not just the likelihood of the observation given the hypothesis but also the prior probability of the hypothesis and of other explanations for the observation. Adding to the confusion is the association of Bayes with the assumption that, prior to making an observation, all potential explanations are equally likely. This idea, called “equal priors” is not the issue. At its core, the disagreement is about the meaning of probability. Is it the long-run frequency of an event or the plausibility of a proposition based on background information?
I believe the best way to understand both the difference between Frequentist and Bayesian viewpoints and the difference between Bayes’s Rule and what Bayes actually wrote is to present two related numerical examples of using an observation to judge a hypothesis. The first is a coin problem that was given to me during a job interview many years ago. The second is the “billiards” problem that Bayes posed in the 1763 essay, published posthumously, that made him famous. In the initial version of each problem, the probabilities of the hypothesis and its alternatives are clear. I will show how Bayesians and Frequentists both would solve the problems using the formula known, somewhat inaccurately, as Bayes’s Rule. Along the way, I will summarize what Bayes actually said. Then, I will change each problem so that the probabilities of the hypothesis and its alternatives are no longer clear. This will allow me to differentiate between the Bayesian and Frequentist approaches. We will see how it ultimately comes down to whether probability is the plausibility of a proposition or the long run frequency of an event